With the development of miniaturized electronic components greatly contributing to the development of convenient human-computer interaction devices, handwriting recognition is widely used in our daily life, such as banking, medical, postal, and legal services. Handwritten character recognition methods are mainly divided into two categories of methods: online and offline recognition. Current online recognition methods scan static images of previously written text files, which are widely used in various fields, such as banking, medical and legal industries and postal services. Optical Character Recognition (OCR), for example, is widely used to digitize text from images of old manuscripts. These methods are image-based or require multiple devices and are costly.
The Tsige Tadesse Alemayoh team in Japan has designed a compact digital pen based on deep learning that enables real-time recognition of 36 numbers and letters. Unlike conventional methods, the smart pen achieves handwriting recognition by capturing the writer's hand motion data through inertial sensors. The prototype smart pen includes a common ballpoint pen ink chamber, three force sensors, a six-axis inertial sensor, microcontroller and plastic structural parts.
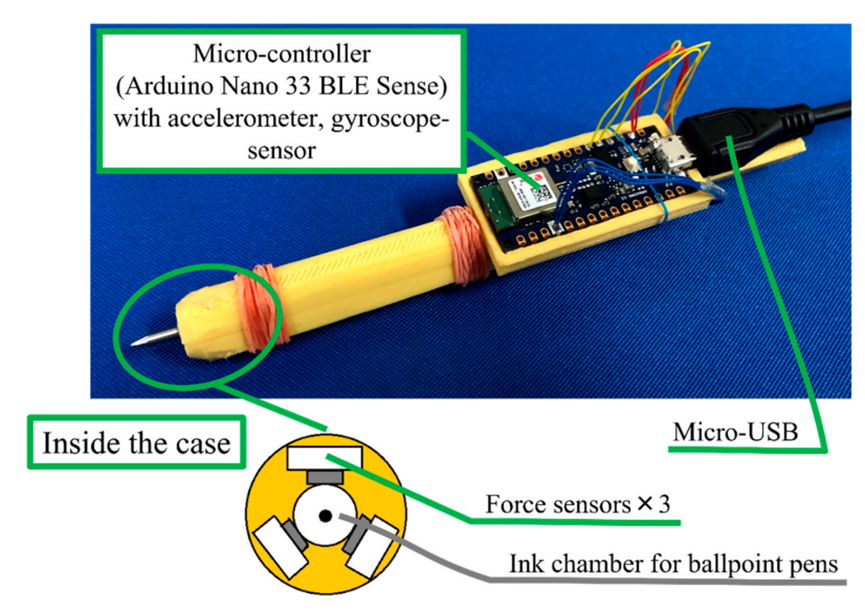
The handwritten data were derived from six volunteers and the data were appropriately adapted and restructured for training using deep learning methods. Four neural networks, visual transformer (ViT), DNN (deep neural network), CNN (convolutional neural network) and LSTM (long and short-term memory), were tested for the training model, with the ViT network yielding the best experimental results. With the combination of IMU and force sensor, the accuracy is up to 99.05%.
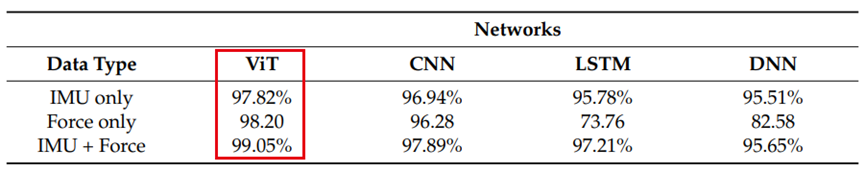
At the same time, the team also used open-source data to validate the trained neural network model and also got good results. As shown in the figure below, the columns represent the predicted letters/numbers, while the rows represent the actual letters/numbers, and the ViT model achieved excellent results in 1000 tests.
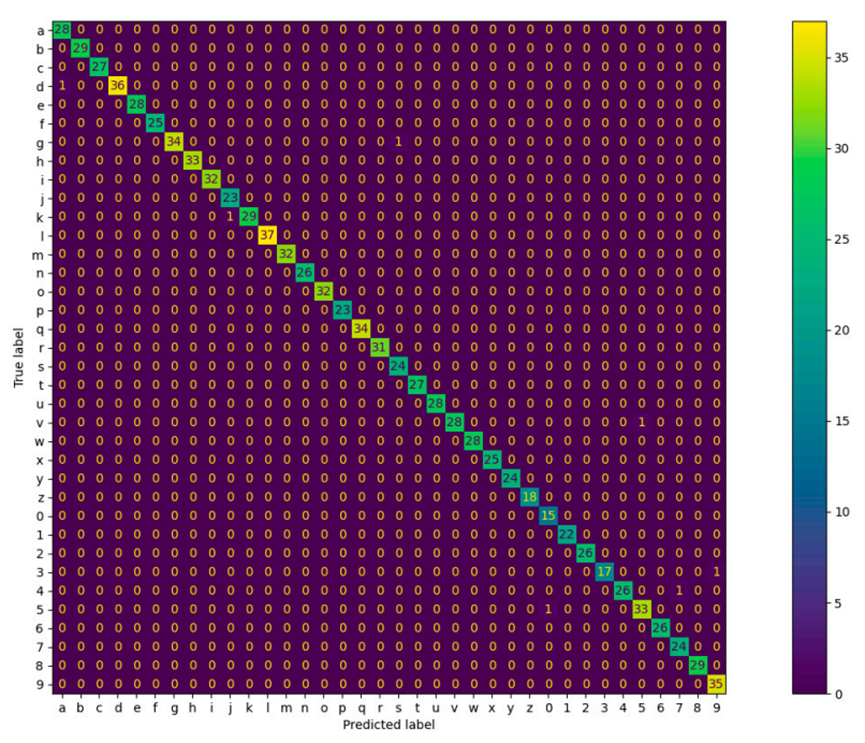
The team says that in the future this approach will be extended to include more subjects, more alphanumerics, and special characters. More methods for structuring datasets and new neural network models will also be investigated to improve performance and eventually achieve a powerful handwriting recognition system that recognizes consecutive handwritten words in real time.
Link to original article:https://www.mdpi.com/1424-8220/22/20/7840